Predicting extreme fire weather using artificial intelligence
A new study published in the Canadian Journal of Forest Research describes a computational model capable of learning that can be used to predict the occurrence of extreme fire weather in northern Alberta, Canada.
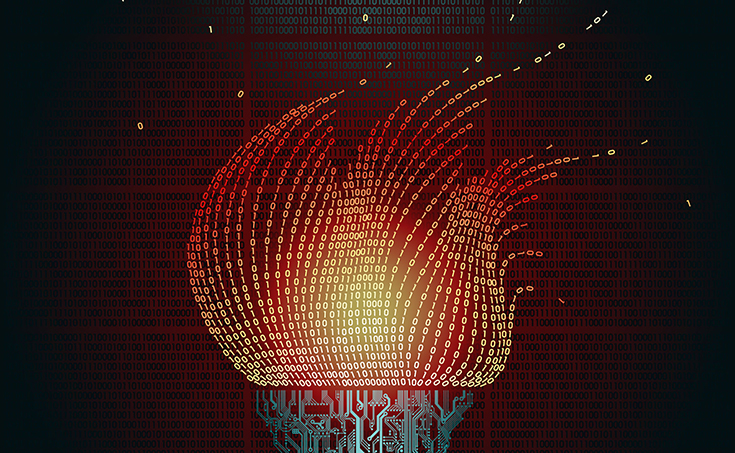
This is the first time that a self-organising map (SOM) has been used to predict extreme fire weather in real time (image: wbraga/123rf)
Developing tools that rapidly predict the potential for extreme fire weather can help fire management agencies ensure community safety by being better prepared and securing necessary resources.
Forest science researchers from the University of Oklahoma and the University of Alberta built and tested the model, referred to as a ‘self-organising map’ (SOM), using atmospheric pressure variables known to affect weather conditions. The model generates maps that predict where and when extreme fire weather is expected in northern Alberta.
“Most of the impacts from wildland fire happen during a short period of extreme fire weather that is hot, dry, and windy,” says Dr Mike Flannigan, co-author of the study and professor at the University of Alberta. As real-time meteorological data is collected throughout Alberta’s fire season from May to August it can be inputted into this SOM model. The model then learns from the new data inputs and generates updated maps and predictions. This process suggests SOMs: “Could be used in day-to-day fire management and as part of an early warning system,” according to Flannigan.
Similar to the human brain, SOMs are described as an array of neurons that can be ‘trained’ to find patterns in data and model complex relationships. SOMs are a type of machine learning system – an application of artificial intelligence whereby machines learn by themselves and not by direct programming. SOMs have been used previously to predict other weather events, such as monsoons, but this is the first study to use this technique for predicting extreme fire weather in real time.
For predicting fires, compared to simpler statistical models traditionally used, an SOM: “May provide a better and more robust method to flag potential extreme fire-weather events,” the authors explain, because SOMs can handle spatial and temporal weather data that are not linearly related to wildfire traits such as intensity and growth rate.
Last year, the Fort McMurray fire in Alberta was responsible for nearly 90,000 people being evacuated, $3.77 billion in insurable losses and a drop in national GDP – the costliest natural disaster in Canadian history.
The article, “Automated Prediction of Extreme Fire Weather from Synoptic Patterns in Northern Alberta, Canada” by Ryan Lagerquist, Mike Flannigan, Xianli Wang, and Ginny Marshall is published in the Canadian Journal of Forest Research dx.doi.org/10.1139/cjfr-2017-0063.