The role of AI and machine learning in public safety
Digital transformation has brought about important changes to public safety. Emerging technologies like Internet of things (IoT) or big data have proved that the sector can prosper in the age of disruption but what about artificial intelligence (AI) and machine learning (ML)? Marta Azevedo Silva from the European Emergency Number Association (EENA) explores the merits of both.
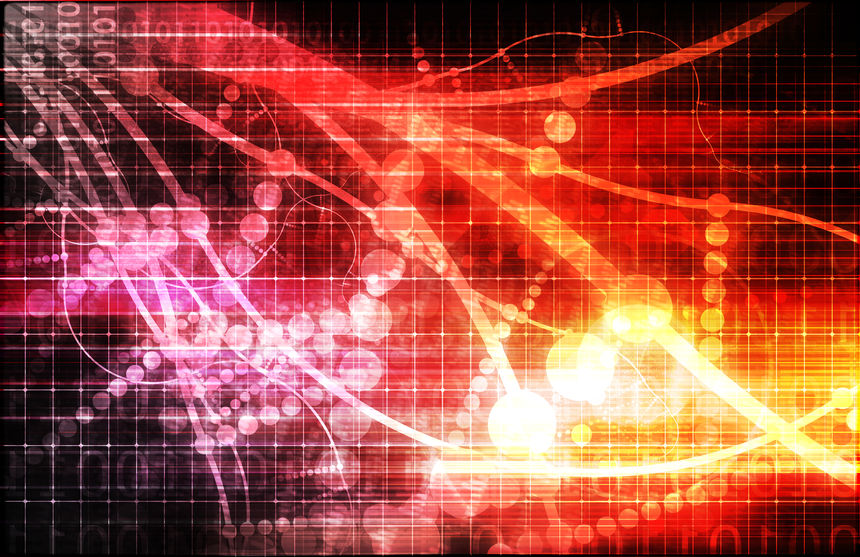
With more and more sectors leveraging AI on a mass scale, one thing is certain: many of us are pondering the pros and cons of this technology. Can AI help to save more lives? How can it strengthen the emergency response field? Image: Kheng Ho
We will start by understanding the basics with a simple question: What is the difference between cats and bagels?
I know what you're thinking – what do cats have to do with a kind of bread? And what does all of this have to do with AI? The following example should explain.
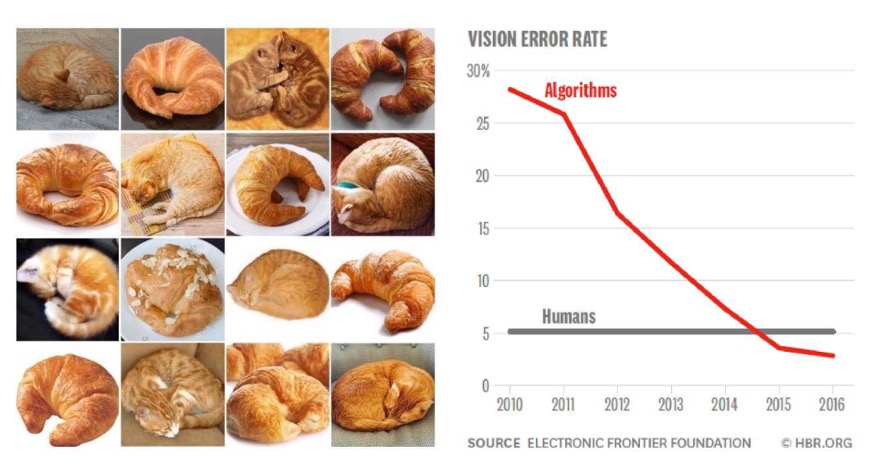
The graph above shows that when distinguishing the differences between images – in this case bagels and sleeping cats – the error rate of humans remains the same over time. However, algorithms learn over time and significantly decrease their error rate
If at the start, algorithms confused similar images, machines are now outperforming humans when recognising objects, with an error rate lower than the human's five per cent rate.
This principle can be utilised in other fields like text analysis or speech recognition. The use of algorithms to automate certain tasks and reduce our risk of error can provide considerable assistance to emergency services.
Before we continue, it is important to understand that this article is not about replacing humans with intelligent machines, but about the creation of a hybrid space where humans and machines can work together to improve the emergency response sector.
When dealing with an emergency, there are numerous external factors that can affect a decision. Physiological and environmental pressure or even emotions can hinder our rationality and affect our judgement in a life-or-death situation.
Machines can process huge amounts of data faster than humans can. They can also analyse a situation with emotional detachment and, as seen above, constantly learn to improve.
Public safety organisations must be open to this new technology, which should be accompanied by a robust ethical framework and comply with privacy regulations.
Artificial intelligence, machine learning and emergency calls
Besides processing data, AI and ML can also find patterns and new insights. Emergency call centres are often swamped with emergency calls and so these tools could help analyse calls and texts, which would ultimately reduce waiting times and save more lives.
According to a recent study, emergency call-takers fail to identify around 25 per cent of cases of out-of-hospital cardiac arrest and consequently lose the opportunity to provide CPR instructions to the caller.
Every year, over 350,000 cardiac arrest cases occur outside of the hospital setting. If CPR is applied within the first minutes, the survival rates increase substantially.
AI could fill this gap and help with the early detection of cardiac arrest, and this is what a Danish company is currently working on. It developed an AI system that analyses emergency calls to predict out-of-hospital cardiac arrests more accurately. The system can detect a cardiac arrest based on historical data. The algorithm listens to the call in real time and will alert the call-taker on the screen if the caller is having a cardiac arrest.
In 2018, EENA launched a pilot project with the company to test how this system could work alongside emergency call-takers in different pilot sites in France and Italy. Machine learning proved to be an important tool to support these professionals. The study concluded that a machine learning framework could augment the capacity of call-takers and dispatchers to identify out-of-hospital cardiac arrest in emergency calls.
Injury diagnosis
An Israeli start-up is using advanced AI technology to help dispatchers to know if a car accident requires an ambulance.
When a car crash occurs, their system creates a medical report in real time with data regarding the forces applied on the passenger, such as duration, moment, vector. This data is generated by their algorithm and sent automatically to the Israeli emergency medical services, Magen David Adom (MDA). This tool can bring first responders to the scene quicker and prevent complications from injuries that otherwise might not be identified.
When a person is in distress, according to this article: "It takes an average of five minutes during the day and seven minutes at night before someone calls for an ambulance." With the MDGo system, the emergency services are alerted in seconds and ambulances can be dispatched automatically according to the type and severity of the occupant’s injuries. With this AI-based technology, the start-up estimates that vehicle fatalities can be reduced by 44 per cent.
Firefighters’ safety
AI can also help firefighters to respond more effectively and reduce exposure to extreme conditions. A Spanish start-up developed an AI-based cognitive health monitoring platform to measure toxins that firefighters are exposed to when battling fires. And a solution developed in Portugal uses visual recognition AI on captured images from surveillance cameras and other data sources to detect fires in forests, natural parks and even mining areas and industrial facilities. This early fire detection is fundamental in containing or reducing damage, monitoring potential risk areas, and shortening reaction times.
This solution was implemented in Europe, the US and Brazil’s Amazon region.
Crisis management
In disaster response, it is crucial to have a good grasp on what a new terrain looks like. AI can help disaster responders to know what resources need to be mobilised. The violent Nepal earthquake in 2015 demonstrated the practical use of AI to spot urgent needs and identify infrastructure damage.
Another organisation has been providing support during several disasters, from man-made incidents to natural hazards, by fostering unmanned systems that are being used effectively by formal management agencies.
Its work and that of other organisations has proved that AI can support relief efforts and change the way emergency teams respond to and prevent a disaster. In a further example, humanitarian aid was deployed with a robot lifeguard to a Syrian boat of refugees arriving to Greece to understand which people needed immediate help. The organisation also explained the importance of AI to help identify which language a person uses and the good use of ML to enhance situational awareness, such as predicting wind, assessing the number of boats that will arrive on the shore, and so on.
Another interesting initiative was conducted by the Humanitarian OpenStreetMap Team (HOT). As part of Microsoft’s AI for Humanitarian Action programme and in partnership with Bing Maps, the team produced AI-detected open building datasets for Tanzania and Uganda to meet the challenge of mapping unmapped areas and improve mapper experience. Mapping is fundamental in disaster risk reduction and integrating deep learning can be valuable when developing a sturdy humanitarian response.
In an emergency, situations change very quickly. Here, just a few examples of how AI can be a game changer for public safety have been highlighted, including helping to get information faster and more accurately, reducing repetitive and procedural activities currently carried out by humans, identifying new trends, or even increasing visibility and understanding.
Implementing AI in emergency response requires great consideration. AI holds a vast potential if applied correctly – considerations about ethics, fairness and compliance with privacy regulations should be prioritised.
AI is not a panacea but it can certainly be a good assistant to emergency services professionals by augmenting and enhancing their work. It is time to stop being afraid of a human-machine collaboration. The technology is already being used in other sectors of society and is getting closer and closer to having a predominant role in the public safety sector.
If emergency services do not make use of these technologies it could lead to opportunities being missed and, consequently, lives being lost.
References:
American Heart Association: CPR Facts & Stats - How CPR is changing (and saving) lives, AHA
Blomberg S, Folke F, Ersbøll A, Christensen H, Torp-Pedersen C, Sayre M, Counts C, Lippert F (2019): Machine learning as a supportive tool to recognize cardiac arrest in emergency calls, Resuscitation 138
Cleve et al (2020): Project Report: Detecting out-of-hospital cardiac arrest using artificial intelligence, EENA
Gomez et al (2019): Artificial Intelligence & Machine Learning in Public Safety, EENA
Leichman A (2019): Now your car can call an ambulance for you, ISRAEL21c
Meier P (2015): Virtual Aid to Nepal, Foreign Affairs